Artificial Intelligence: The State of Play
The accelerated adoption of artificial intelligence across an increasing number of sectors during the coronavirus crisis means it will soon become a general purpose technology. But critical improvements that it may bring, including for sustainability, will not come solely from AI but from the understanding of, and engagement with, how the technology interfaces with the existing economic, environmental, social and political world, write Marjorie Buchser and Matthew Oxenford.
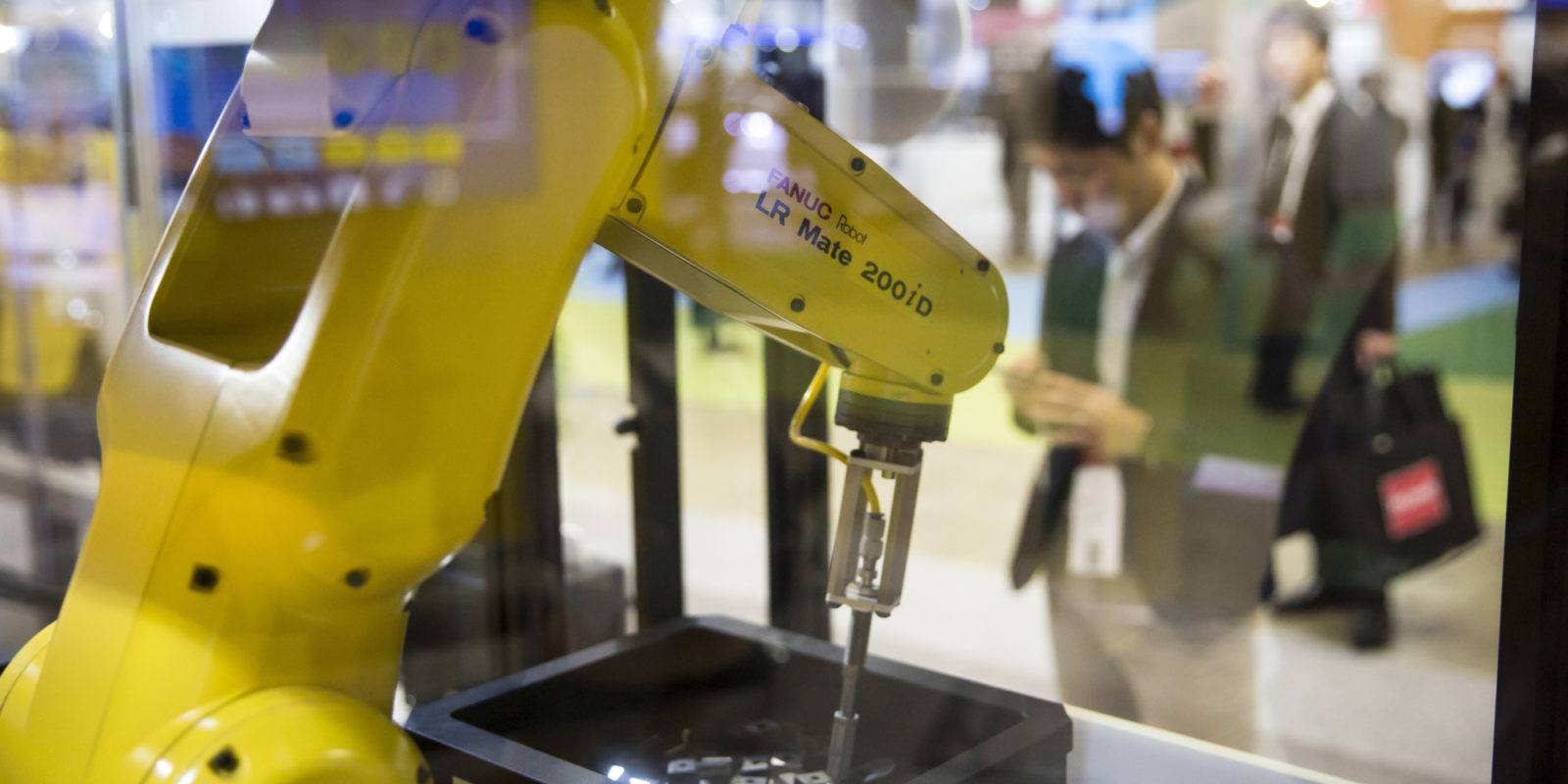
A robotic arm demonstrates an AI system at the Ceatec Japan 2018 consumer electronics show. Image: Tomohiro Ohsumi/Getty Images.
There is currently no unified definition of what constitutes artificial intelligence (AI). On a fundamental level, artificial general intelligence (AGI) – also called ‘strong’ or ‘broad’ AI – is defined as general purpose systems that replicate or even exceed the human mind. While experts differ on how distant this breakthrough is, it is most likely still some decades away.1
Instead, research and applications refer to ‘narrow’ AI – also called ‘weak’ AI – which includes domain-dependent and problem-specific algorithms that can achieve results on defined cognitive tasks at a level matching or exceeding those of a human.2
In the last decade, the most promising area of research has been in machine learning: developing systems that can learn how to perform a task defined within a set of parameters without using explicit instructions by relying on patterns and inference and iterating more sophisticated versions of themselves that perform increasingly well.
The particular innovation of ‘deep learning’ – a subset of machine learning that utilizes a hierarchy of machine-learning algorithms where higher levels of abstraction are built on lower ones in a way that typically utilizes artificial neural networks3 – has allowed for an unprecedented number of breakthroughs.4 While traditional programmes develop analysis with data in a linear way, the hierarchical function of deep learning systems enables machines to process inputs in a non-linear fashion.
Many of the recent advances in machine learning have made use of large quantities of standardized digital data – usually ‘structured data’ that a machine can learn from by identifying the patterns within. The existence of these large-scale, ever-growing, varied datasets – ‘big data’ – has only been possible since the wide adoption of the personal computer and the internet and the subsequent explosion in digital content created in the last 10-20 years.
Although most machine learning systems utilize this wealth of data extensively, the terms ‘artificial intelligence’, ‘machine learning’ and ‘big data analytics’ describe overlapping but distinct processes. In fact, not all analytic processes that use big data make use of machine learning.5678 Indeed, similar overlapping definitions plague other related sectors such as robotics, autonomous vehicles and the internet of things or smart machines, all of which, have become more sophisticated with the most recent advances in the field of AI.
Generally, when AI has been discussed in a commercial or industrial sense since 2015, this has been about advances in narrow AI that has been brought about by machine learning and deep learning in particular.
AI adoption
The ambiguities in defining AI also mean that estimates as to the size of the AI sector vary significantly. Yet, experts generally agree that it should take on the features of a ‘general purpose technology’ (GPT)9 – a technology that enables the creation or revolution of new technologies and industries across sectors throughout the economy. AGI, almost by definition, has the potential to become general purpose as it promises, to develop artificial versions of human cognition that are clearly found across the range of human activities.
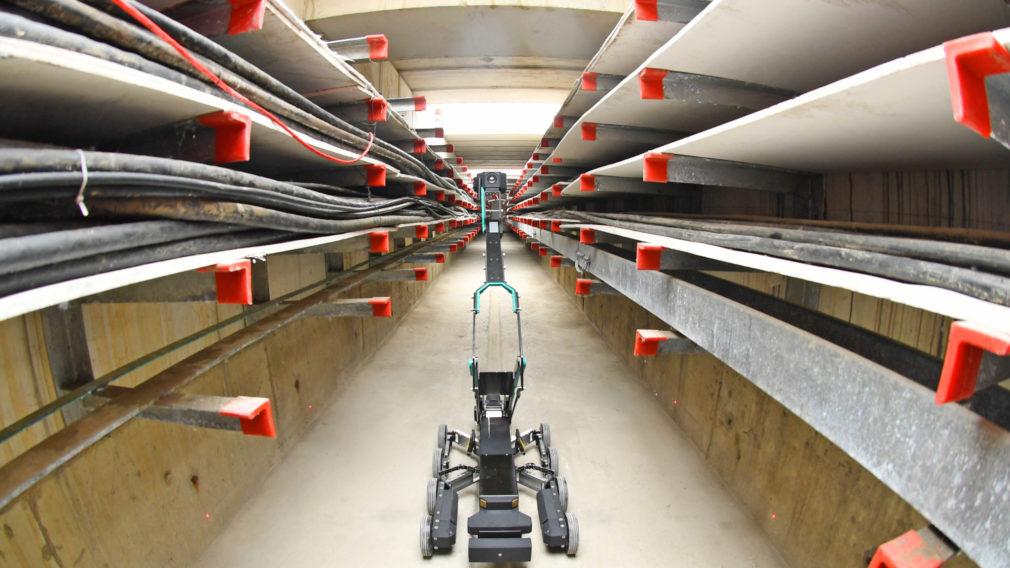
An intelligent robot inspects the equipment at a 1,000 kV ultra-high voltage (UHV) electrical substation in Huainan, Anhui Province of China. Image: Song Weixing/VCG via Getty Images
Prior to the pandemic, KPMG estimated that total AI spending by enterprise in 2018 was $12.4 billion but set to grow to almost $232 billion by 202510 while the International Data Corporation estimates that worldwide spending on ‘artificial intelligence systems’ will reach $79.2 billion in 2022.11 Although these studies measure subtly different things with slightly different methodologies and achieve incredibly divergent results, what is constant across them is the potential for exponential growth in the sector, however broadly it is defined.
The health crisis is likely to accentuate this trend and further accelerate the adoption of AI systems across industries. According to recent analysis from McKinsey, developed countries vaulted five years forward in consumer and business digital adoption in just eight weeks during spring 2020.12 Companies have been forced to digitize parts of their business to protect employees and serve customers facing mobility restrictions. Communities around the globe have radically altered their social, political and economic practices, shifting many of their activities online. This rapid migration to the digital world will not only fast-forward the deployment of AI but will also, over time, offer more accurate structured data for AI systems to be trained on.
Past GPTs may offer relevant insights on the future of AI and it is worth reviewing some of their key characteristics. Examples of GPTs include steam power, electricity, the internal combustion engine, the personal computer and the internet. Like most new technologies, they are implemented in an ‘s-curve’ fashion – being adopted slowly at first as the costs of adoption are high and the uses it can be put to are limited and then speeding up as costs drop and applications start to proliferate until only a tiny minority of holdouts remain not adopting the technology. As a result, they are first deployed in limited fashions within existing systems before being applied in more innovative ways across more sectors.
Second, the bulk of productivity gains and industrial transformations are not realized often until decades later. A classic example of this phenomenon is the transition from steam-powered factories to electric motors in the late 19th century whose introduction into industry has drawn parallels with AI from contemporary thinkers.13
Electric motors were more flexible and portable than steam-powered motors which had to be organized around one central shaft. However, the process innovations that this allowed, such as the assembly line, were not themselves immediately obvious and took decades to be realized.14
Similarly, the rise of personal computing began as early as the 1970s in business and the early 1980s for personal use. However, related gains in productivity were not realized until after the mid-1990s as the innovations that led to improved business practices and the development of the internet took over a decade to be developed and scaled to sufficient size to show up in productivity statistics.15
At the end of 2019, AI, and machine learning in particular, was still in the early phases of adoption. Assuming that it would follow the patterns of GPTs, it was expected that the gap between the potential productivity gains and actual implementation of AI across industry would be vast and take decades to be fully bridged.
However, in data-rich sectors, this timeframe may have been significantly shortened by the COVID-19 crisis and subsequent boost in R&D investments. Take the healthcare sector for example. The speed and scale at which researchers launched efforts to develop a vaccine were remarkable, and in part enabled by AI-powered innovation. Digital technologies are now being deployed in all aspects of healthcare with a sharp increase of data sharing efforts across organizations. AI is directly informing emergency responses and used to predict and prevent potential outbreaks.
Even in a context of accelerated digitalization where technology deployment is broad and affects most industrial sectors, AI penetration could remain uneven for years to come, making the most headway in areas that require the least revolutionary change to adopt it.
In surveys of several industrial sectors done by Accenture in 2018, over a third of firms had employed AI in some capacity but most commonly in areas that were already data-rich such as streamlining the existing interactions between computers and machines or data or in data-intensive processes such as finance or analytics. In each sector, the development of new processes – the most transformative use of AI – was the least common application making up less than 10 per cent across industry.16 17
Prior to the pandemic, studies also highlighted that the industries that already spend significant sums on R&D were also likely to be the first to adopt AI in significant quantities. All the 10 largest corporate R&D spenders worldwide are in the technology, automotive or healthcare sector and, not coincidentally, these sectors were among those expected to adopt AI the fastest.19
Early evidence suggests that digital technology adoption during – and following – the coronavirus pandemic will continue to follow this predictable path of prioritizing low-hanging fruits. So far, most R&D-intensive industries have successfully adapted to new requirements, rapidly pivoting towards digital business models. In a poor year for most companies, a minority of tech-focused sectors have bucked dismal trends including healthcare and pharmaceutical groups boosted by the search for a COVID-19 vaccine and technology giants bolstered by working from home trends.20
While COVID-19 has buoyed uptake of digital technologies in some sectors, heavy industries like cement, steel and chemicals manufacturing have, to-date, continued to lag. The coronavirus pandemic could further exacerbate this gab as budgets are squeezed in the face of economic downturn. Already, engineering, construction and building material (ECB) companies have experienced significantly larger stock price declines than average since February 2020,21 while within the chemicals sector, year on year spending on R&D has declined, in some cases, by more than 10 per cent, a trend that is expected to continue across industrial sectors throughout 2020.22
AI implementation across heavy industry by processes
Source: Accenture (2018)
Stalled R&D spending in a sector already slow to adopt digital technologies could have a significant impact on climate trajectories and sustainable development targets. Heavy industry accounts for some of the highest emissions of greenhouse gases – almost 30 per cent of CO2 emissions globally – and AI systems could have a significant role in its decarbonization. While existing applications provide optimizations with modest emissions savings as their by-product, much more radical emissions savings could be achieved through a more holistic use of AI in heavy industries, from material discovery to manufacturing, use and at end of life, yet these are also the least deployed. AI-driven innovations could help increase efficiency, accelerate decarbonization and ultimately redesign industrial processes for a green transition.
The role of the state in stimulating innovation
Over time, as with other GPTs, process innovations will eventually drive adoption and structural changes in all industries. When AI becomes widely implemented, the idea of a separate AI sector should become nonsensical – similar to describing manufacturing or ICT as part of the ‘electrical sector’ simply because they use electricity. Integration will be such that firms – except for the manufacturers of the basic hardware and software – will interact with the technology understanding only its applications and not necessarily its underlying technical specifics.
While much of the adoption of AI systems in the last months has be driven by COVID-induced digitalization, governments have also had a significant role to play in this transformation and, in recent years, have increased their efforts to develop competitive national AI markets.
As a result, over the last two years, at least 35 countries have established some form of AI strategy, task force or policy framework.23 There is also appetite for international cooperation on the use of AI to ensure it is utilized transparently and for the common good of society.24
The amount of money committed to national plans varies greatly – from $12.5 million per year in estimated spending by Russia 25 in 2018 to €1.5 billion annually committed to AI training in the French plan with €700 million invested in AI research.26
However, given the large and relatively homogenous domestic consumer markets, the size of their existing AI industry and research networks, the cultural receptiveness of their consumers to sharing data and their governmental and military buy-in, the countries whose AI strategies will have the most impact globally are likely to be China and the United States.27
These countries have taken somewhat contrasting views in developing AI so far. China’s New Generation Artificial Intelligence Plan adopted in 2017 is among the more comprehensive and ambitious national AI strategies published.28 The plan heavily focuses on outlining priorities for basic research in AI and creating ‘AI Innovation Heights’ in China. However, it also sets out priorities for expanding AI’s application in industry as well as social priorities such as healthcare, education, government and environmental protection where it envisages ‘an intelligent monitoring large data platforms and systems covering the atmosphere, water, soil and other environmental areas.’
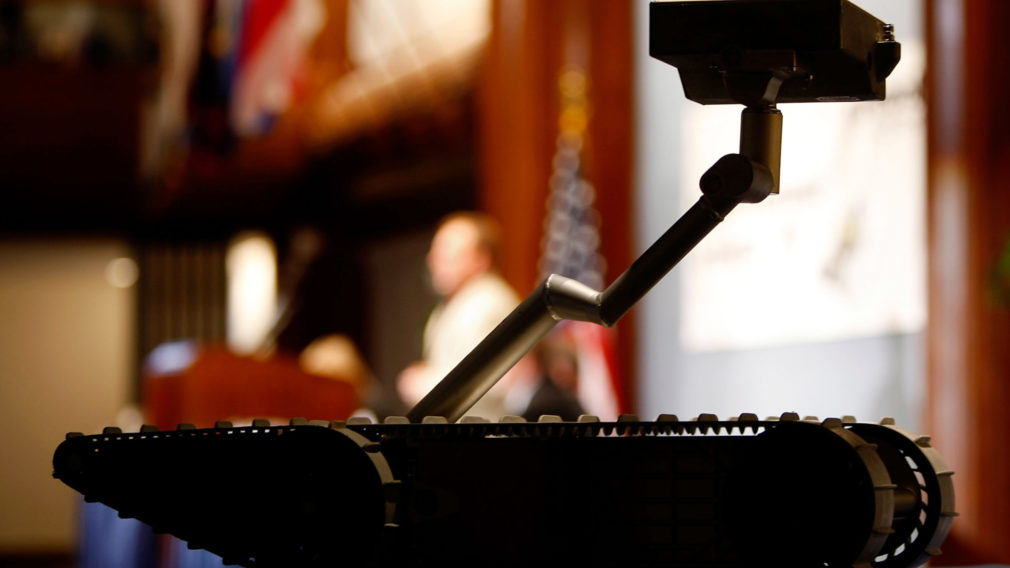
A Small Unmanned Ground Vehicle, or FCS SUGV, during a demonstration by iRobot representatives of 'life saving military robots' in Washington DC. Image: Win McNamee/Getty Images.
While both the Obama and Trump administrations have articulated plans for AI, the United States has always been politically opposed to committing to formal industrial policy. Instead, the US government is providing significant funding for its AI policy through the military – the Department of Defense (DoD) spent $7.4 billion in unclassified research into AI and related fields in 201729, and in 2019, the DoD released a formal strategy for AI deployment called 'Harnessing AI to Advance our Security and Prosperity'.30 Furthermore, in February 2019, the Trump administration signed an executive order directing US government agencies to prioritize AI spending in R&D to support AI-conducive regulation and to support the training of AI scientists.31
However, what will be critical in the long-term deployment of AI across industry is not only the support for research, and the distribution of the technology itself, but the ability for entrepreneurs to tinker and innovate, applying breakthroughs to adjacent fields and combining AI with other technologies. This critically includes recognizing how advances in AI might unlock productivity advances in adjacent fields that may not be inherent in AI itself.
The ability of countries to support this is not necessarily linked to their ability to support basic AI research. By way of historical analogy, many of the first internal combustion engines were developed in Europe by inventors, such as Jean Lenoir of Belgium and Nikolaus Otto of Germany, but it was the technologically unrelated invention of the assembly line, pioneered by American Henry Ford, that allowed automobiles to be produced cheaply and become ubiquitous.
The development of AI may be revolutionized by similar larger ecosystems and process innovations. It is these leaps from basic AI research to real-life, scalable applications that will determine where the leaders in AI will be found. While the support for entrepreneurship in AI and related fields is a component of many national plans, including China’s, the ability of these lateral transfers to take place is still very much in its infancy.
Implications for sustainability
As demonstrated above, AI applications for heavy industries and its adaptation for sustainability purposes has been limited. Insofar as industries have adopted AI within the context of sustainability, it has been for optimizing existing processes such as minimizing energy use in data centres or warehouses, minimizing fuel consumption or similar optimization strategies.
Furthermore, environmental responsibility remains largely absent of ethical concerns regarding AI systems. So far, most AI national or industrial strategies issued by governments focus primarily on harnessing the economic potential of AI. Other sets of principles – such as the Future of Life Institute’s Asilomar Principles32 – while encouraging AI to be developed with an eye towards safety and social benefit do not include sustainability principles.
In early 2020, researchers from the Berkman Klein Centre attempted to map the ethical and right-based approaches included in 36 national strategies, intergovernmental frameworks and corporate declarations. While the authors noted the significant impact of AI on the environment, they did not find sustainability to be a prominent theme. Less than 20 per cent of all documents addressed, directly or indirectly, environmental responsibility and the necessity for those who build and implement AI technology to be accountable for its ecological impacts.
While the potential for AI to completely reinvent industrial processes globally has yet to be realized, the COVID-19 crisis could be one such juncture that sees increased application of AI for sustainability. For example, the reduction in manufacturing output during lockdown phases of the pandemic highlights the need for heavy industries to reorient existing business models and implement systems that increase resilience to similar shocks in the future while also providing a moment to invest in technologies that will increase their decarbonization potential.
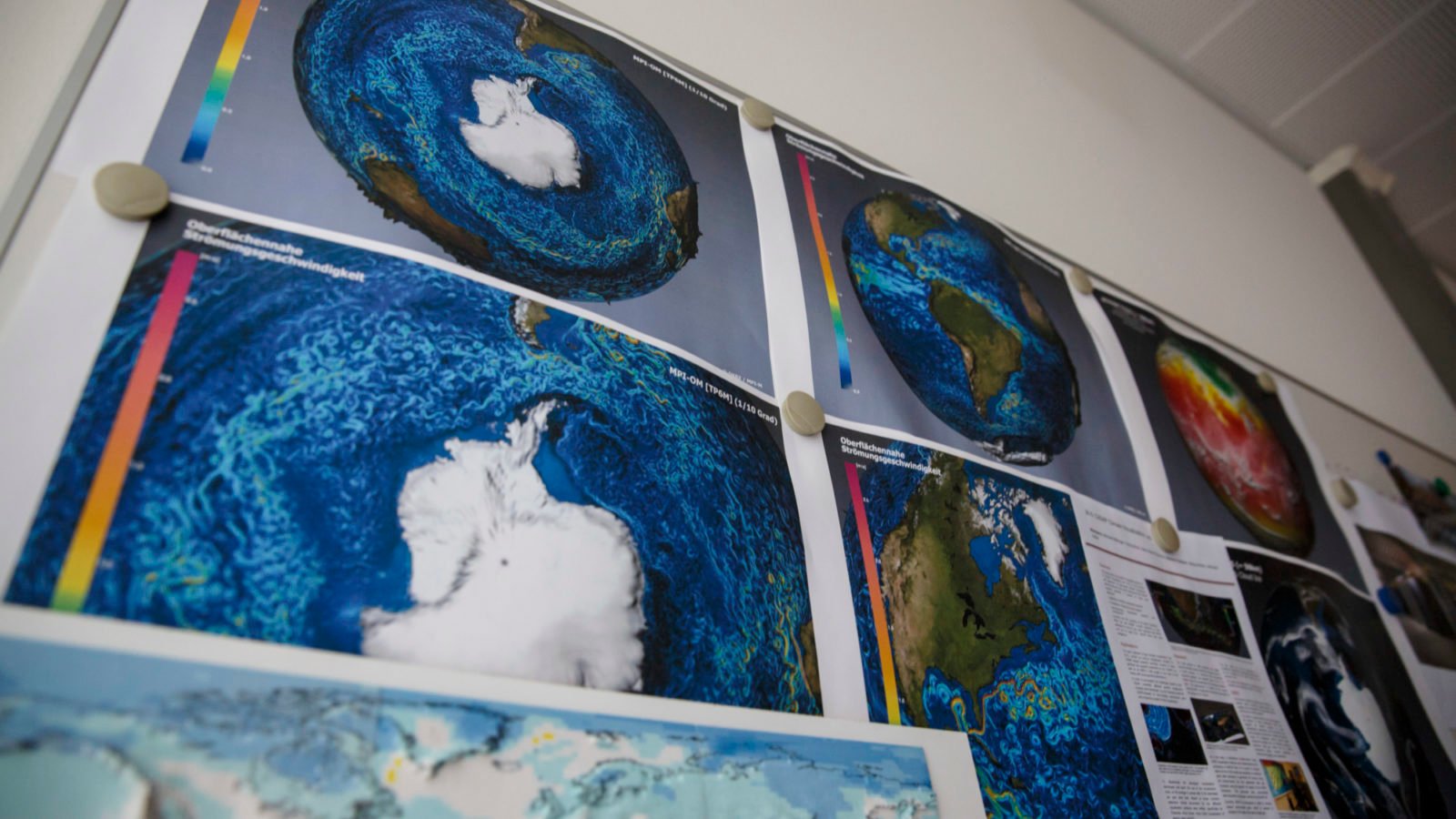
Renderings of a "Mistral" supercomputer are seen at the German Climate Computing Center (DKRZ, or Deutsches Klimarechenzentrum). The DKRZ provides HPC (high performance computing) and associated services for climate research institutes in Germany. Image: Morris MacMatzen/Getty Images
Governments, under pressure to ‘build back better’ amid the economic impact of the COVID-19 pandemic, have made some commitment to funding decarbonization technologies. In the UK, the government committed £350 million for technologies to decarbonize the ‘hard to abate’ heavy industry sectors. This package includes £149 million combined government-private sector funding to drive innovative low-carbon materials use and increase the use of AI and robotics and £10 million for state-of-the-art construction technology such as digital twins.33
The EU’s economic recovery fund of €750 billion could see up to €150 billion to fund sustainability-focused outcomes while its revamped 2021-27 budget of around €1.1 trillion has committed 25 per cent to climate policy. However, the specific funding allocation to AI for sustainability within these budgets is less clear. On top of this the European Commission’s Innovation Fund commits up to €10 million to 2030 to funding highly innovative technologies that can significantly reduce emissions.34
The European Commission’s funding goes some of the way to supporting the ambitions of their European Green Deal, which has pointed to the breadth of applications of digital technologies to monitor air and water quality, to manage resource use and combat climate change while emphasizing the importance of interoperable data to both understand and tackle the environmental challenges we face.35 The European Commission’s white paper on AI has more specifically highlighted the critical role AI and digitalization in delivering the ambitions of the European Green Deal and in enabling business development for the green and circular economy.36
The development of new principles for AI that include a sustainability element may encourage the private sector and governments to think beyond the existing issues of productivity, efficiency and social inclusion. Whether new innovation comes from the civilian or government sector, AI has the potential to leave the narrow confines it has been implemented in so far and be more comprehensive.
But, the subsequent improvements AI may bring, including for sustainability, will not come solely from experts in AI technology but from understanding and engagement with how the technology interfaces with the existing economic, social and political world.
This article was written with the generous support of the European Climate Foundation and DeepMind Ethics and Society.