Harnessing Artificial Intelligence to Decarbonize Industrial Sectors
While AI applications to decarbonize heavy industries are promising, a focus on process optimization over its more radical use could limit its potential. However, establishing knowledge bases and policy frameworks to foster innovation could speed up the application of AI to disrupt business-as-usual operations and deliver decarbonization.
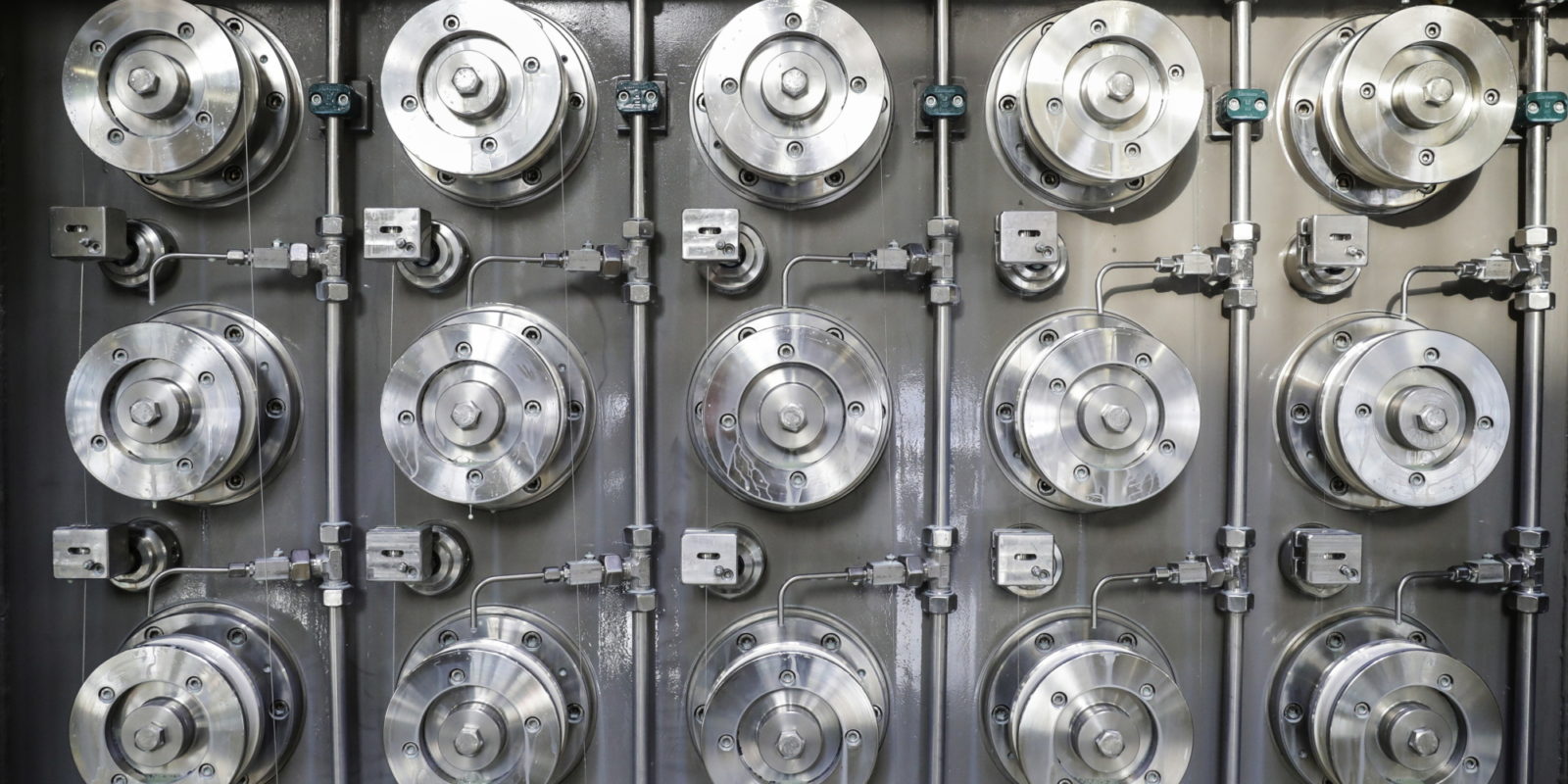
Rolling wires made from precious metals at a non-ferrous metals plant. Image: Kirill Kukhmar\TASS via Getty Images
As global use of metals, fossil fuels, sand, limestone and gravel are forecast to more than double between 2011 and 2060,1 heavy industrial sectors are under increased scrutiny to take more significant steps towards reduced material consumption and decarbonization. Undoubtedly, the COVID-19 pandemic has hit production of cement, steel and chemicals processing: cement production was, globally, 4.4 per cent lower in the first quarter of 2020 than 2019.2 Yet such reductions have not significantly challenged estimates that these ‘harder-to-abate’ sectors account for almost 10 Gt or 30 per cent of total global carbon dioxide emissions annually,3 and may increase to 46 per cent of total global emissions by 2050.4
In order to tackle this challenge, heavy industrial sectors are already adopting renewable energy and efficiency measures. Numerous steel manufacturers are pursuing hydrogen-powered production processes while the cement sector has started to turn to photovoltaics (PV) and concentrated solar power (CSP) to increase renewables into its energy mix.5 Aluminium company, Norsk Hydro, and cement producer, Cemex, among others, signed up to renewable-energy power purchase agreements.6 Other sector actors, like the Swedish steel producers making up the BRIT collaboration, are investing in renewably produced hydrogen for steel production plants and processes.7
However, wide-scale adoption of renewable sources within the industrial sector has been somewhat limited – estimates suggest that they accounted for just 14 per cent of industry’s energy use in 20158 increasing to just 14.5 per cent in 2018.9 The health crisis and looming recession may, however, have altered this trajectory and commitments to decarbonize industrial sectors through economic recovery packages could increase uptake.
Circular economy business models also have the potential to cut emissions from aluminium, plastics, steel and cement by 40 per cent globally and by 56 per cent in more developed economies by 2050.10 Despite this potential, rates of materials recycling remain stagnant – the Global Circularity Metric estimated 8.6 per cent global circularity in 2020.11 Aluminium production is a case in point: recycled aluminium scrap makes up around just 20 per cent of total primary production. Uptake remains low because adopting circular economy approaches requires a consistent supply of scrap provided through effective materials processing and redistribution across the board – from building demolitions to domestic waste.
While the potential to deliver decarbonization and resource efficiency within industrial sectors has not yet been met, the application of digital technologies from the new industrial revolution could have a significant impact in reshaping industrial value chains. Within this context, AI and related technologies – such as big data analytics, sensors and the internet of things (IoT) – could transform the industrial sector well beyond process or domain-specific optimization and radically disrupt the supply chains of specific materials.
Domain-specific optimization
Using AI to increase efficiency gains has already demonstrated significant potential that can be applied in industrial sectors. Notably, Google’s DeepMind implemented a machine-learning system within a Google data centre to make significant gains in efficiency. Utilizing historic data collected by sensors, it successfully applied a machine-learning neural network to decrease the amount of energy used for cooling servers by up to 40 per cent in 2016.12
The likes of Element AI have already implemented similar variance-analysis techniques to compare energy usage on high- and low-performing days within industrial settings. Such applications help to identify changes required and investments needed to reduce fuel consumption and emissions through industrial processes.13
Companies are also making headway in applying AI to manage uncertainties and reduce inefficiencies within specific production practices. For instance, in manufacturing, the deployment of anomaly detection models can quickly identify defects that would otherwise lead to wasted products and rework, thereby reducing material consumption.14 In a similar vein, Yandex Data Factory's work with Russian company, MMK Steel, reduced ferroalloy consumption by an average of 5 per cent through the analysis of historical datasets.15
Other innovations in AI applications could see the decarbonization of entire processes within production. Within the cement sector, a combination of machine vision and CSP have been deployed to optimize the position reflective mirrors to capture the most of the sun’s rays.16 This has provided proof of concept that AI-enabled solar-powered furnaces can reach over 1,000oC. Deployed for commercial use and scaled, solar furnaces could deliver emissions reductions from the carbon intensive calcination process.
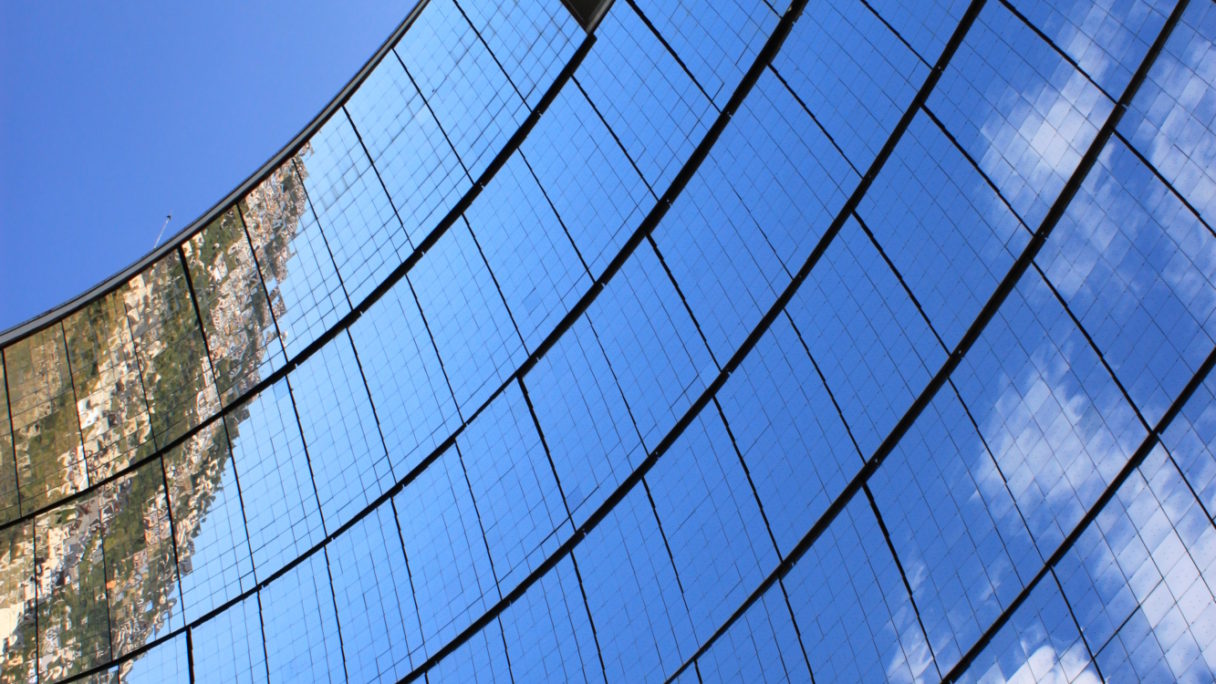
One of the largest solar furnaces located in France. Image: Ruti Robles / Getty Images.
Yet, when looking to deliver more significant optimization of industrial processes, AI is only as good as the data at its disposal. Existing data collected may be incomplete, lack granularity or fail to address gaps and inefficiencies in the system that could have the most significant impact on resource-use reduction. As such, data collection that is only driven for the purpose of economic savings or productivity gains can severely limit potential carbon reductions. AI’s potential to deliver deep decarbonization requires heavy industries to establish stronger data readiness and to specifically refocus data collection strategies on to decarbonization.
While the uptake of disruptive technologies is expected to grow exponentially across the board, adoption within heavy industries is expected to be below average by 2022.17 Chemicals and materials processing have been demonstrative of this point continuing to lag behind market leaders,18 a trend that may be perpetuated by the COVID-19 pandemic: R&D spending declined by more than 10 per cent in the chemicals industry in Q1 of 2020 versus 2019.19
Importantly, a lack of ambition to address decarbonization in its own right means heavy industries may fail to fully utilize the potential of AI to shift beyond domain-specific optimization towards supply-chain disruption. ‘Light’ decarbonization through process optimization has the appeal of causing minimal disruption to existing production practices, low implementation costs and immediate yield improvements to boot.
In contrast, while exact estimates vary, the cost of innovative solutions required for deep decarbonization could see up to a 20 per cent increase for the cost of steel and up to 115 per cent for cement and concrete. This could prove a significant barrier to the uptake of disruptions across the supply chain.2021 Institutional inertia from powerful incumbents could, therefore, threaten the scaling up of AI beyond solutions that maintain business-as-usual operations.
Supply chain disruption
AI could deliver more radical disruptions across industrial supply chains for greater decarbonization potential. AI has been applied in the realm of material science in order to simulate scenarios and predict properties of novel materials: AI systems are already up to 30 per cent faster than existing trial-and-error approaches.22
Using AI to identify novel materials can also be harnessed to identify resource use and select materials that best meet sustainability criteria. For example, The European Space Agency funded a project to conduct research into developing new, more efficient alloys that also met certain sustainability principles.23 Utilizing AI algorithms to analyse vast datasets of existing materials, researchers set out to test new alloy formulations including their predicted impact on resource depletion, greenhouse gas emissions and acid rainfall – meaning partners could feasibly calculate the environmental impacts for the new alloy formulations.24
In order to scale up AI’s potential, efforts in materials science need to continue to be trained on knowing the footprint of a given material (e.g. carbon and water) as well as the footprint of its supply chain. This type of innovation could push the industrial sector to substitute existing materials and processes in a way that could unlock wide-scale decarbonization – dependant on the potential resource footprint of a specific material and its supply chain – and enable the adoption of this change at a rapid rate.
However, opportunities for material substitution and disruption along the supply chain must also account for social implications unforeseen by the ‘structured’ nature of material science. While AI might increase the speed at which new, more sustainable, materials can be discovered and produced, the unintended consequences of cobalt mining – from the use of child labour and dire heath consequences for miners to localized water pollution from run-off – bring into focus the need to mitigate potential knock-on effects that AI might induce.
AI can also deliver more effective circular economy business models across the supply chain in order to slow down and narrow options for material consumption. Predictive maintenance, for example, can extend product life meaning that the material input to make new products is reduced.
Furthermore, AI can also be implemented to track materials in order to re-manufacture and re-enter them into the supply chain. Machine learning, in particular visual identification systems, are being adopted to improve rates of municipal recycling where intelligent sorting machines are able to better identify materials and could, in the future, identify material composition.
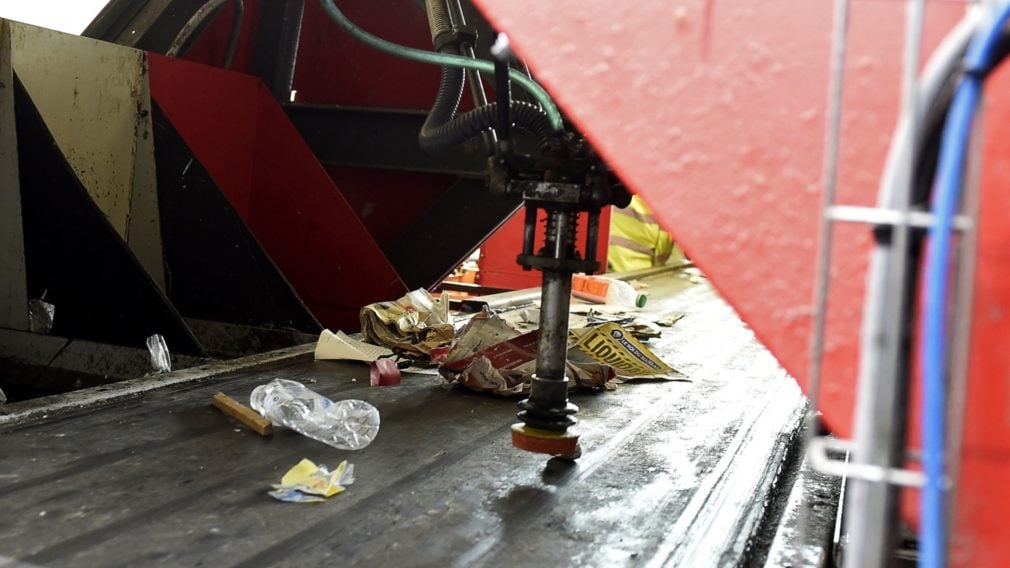
Autonomous AI robot identifies recyclables and other items for recovery. Image: FRANCOIS LO PRESTI/AFP via Getty Images
Digital technologies could also ensure the effective implementation of material passports: individual identities given to materials within the built environment with information on the quantity, quality, and location of urban material ‘banks’ or ‘mines’. It is expected that technologies like blockchain and visual learning will provide the basis of further innovation providing effective management and redistribution of materials where supply and demand have been identified as well as ensuring that product-life extension is built into business models.
Collaborative practices
Data sharing could enable more effective resource allocation and redistribution across the supply chain. Increased transparency and traceability could ensure more effective management of industrial resource use, incorporating data inputs from across a product’s lifecycle, for example, to optimize stock replenishment. Digital twins, whereby exact replicas of physical assets are digitally recreated in order to test different scenarios and conditions, can be used to predict the most promising modes of operation for decarbonization which, if interoperable, could be applied across supply chains.
Fragmented supply chains and data silos reduce the potential for integrative end-to-end applications of AI. There is a need to align the huge array of inputs and actors along the supply chain while also ensuring an ecosystem of trust that will enable open data sharing from diverse and competing companies that also recognizes data sovereignty and security threats, for example, through data-security frameworks.
Recommendations
Disruptions can be difficult to predict and focus on process optimization can stifle step change towards industrial transformation. Existing risk-averse financial models can punish industry leaders while there is lack of robust policy frameworks to drive change. The landscape for facilitating deep decarbonization within the industrial sector needs to provide a framework in which heavy industries can better harness disruptive technologies and balance risk and reward for actors leading the way driving applications that goes beyond process optimization. While COVID-19 recovery packages have begun to make moves to drive industrial decarbonization, more coherent policies are required:
Demonstrating the potential and supporting the growth of AI technologies could help to sidestep incremental approaches that fail to deliver the needed rates of decarbonization. As industry incumbents are increasingly exposed to innovative solutions or challenged by new disruptors, the hope is that they will be driven to adopt or restructure to more radical production business models that dramatically reduce emissions.
This drive for the innovative use of AI could also come from shifts in public sentiment as demand for action and transparency on environmental and sustainability issues increases. Consumers have the power to push companies to question their existing practices and reorganize their supply chain as well as to steer demand towards more sustainable resource use through a cascading process that requires actors along the supply chain to align themselves with consumer wishes.
Provided the enabling conditions are in place, the COVID-19 pandemic could increase the pace of AI adoption. Radical shifts online, both by companies and individuals, demonstrate the viability of transformations to business practices. At the same time economic disruption may push companies to increase digitalization, including AI, in order to remain economically viable in the face of future lockdowns. Here, aligning the digital and green agendas will be vital.25
AI alone will not solve the problems of decarbonization – it must be aligned with other tools to drive the industrial sector to act. Radically reducing emissions from cement, steel and chemicals processing requires supporting political, economic and financial frameworks in order to deliver on AI's potential. Establishing an enabling environment for AI while also shaping narratives around industrial decarbonization will mean that AI can be better applied as a specific solution to help deliver decarbonization within heavy industry.
This summary is based on a workshop hosted by Hoffmann Centre for Sustainable Resource Economy with the generous support of DeepMind Ethics & Society.